The image data are trained through the convolution neural network. The input is given as the feature-based image which separates the weed and plants in the segmented image. The convolution operation is performed on the matrix of pixels and trained through extracted features. The two layers involved are fully connected and pooling layers. The pooling layer helps to oversimplify the output of convolution layers. Both classifiers work well and extract weed infected region accurately, details are given in Table 3.2.
Serial number | Accuracy of weed infected area by neural network (%) | Accuracy of weed infected area by SVM (%) |
---|---|---|
Image 1 | 89 | 94 |
Image 2 | 90 | 92 |
Image 3 | 91 | 91 |
The weed affected region in plant is identified through fuzzy logic as shown in Figures 3.5–3.7.
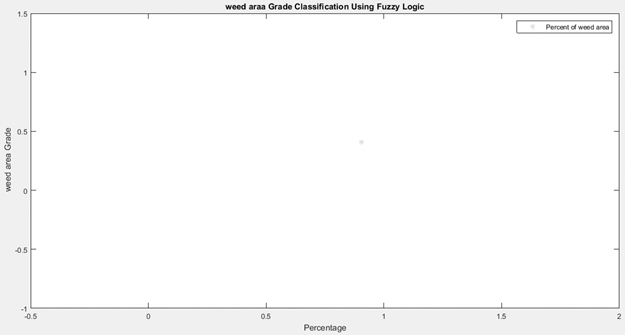
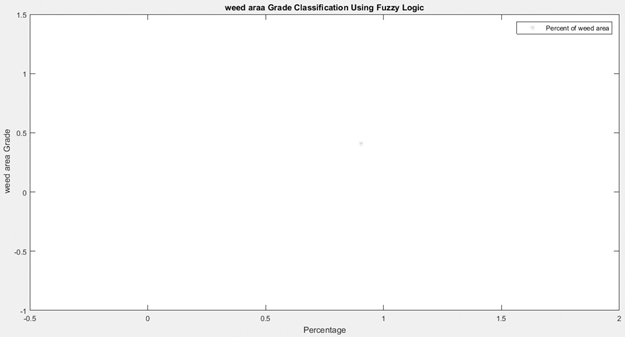
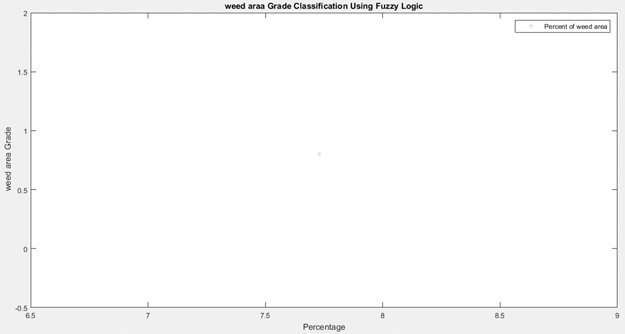
The results of differentiation between weed and plants are shown in Figure 3.8. The bounding box represents disparity between plants and weeds. The differentiation between weeds and plants is done using machine learning algorithms. Such automatic detection methods are used in smart agricultural fields.
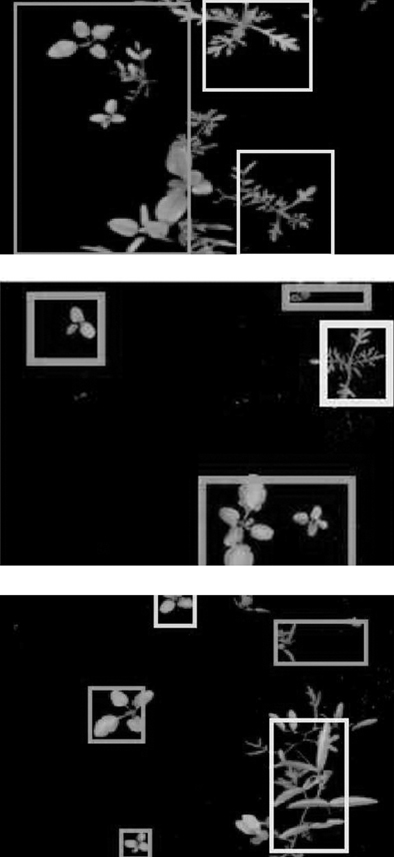
Leave a Reply