Consider two sets of variables that are collected in vectors X and Y, respectively, and imagine that we would like to study the relationship between the two sets. One way for doing so is by forming two linear combinations, Z = aTX and W = bTY, in such a way that the correlation ρZ,W is maximized. This is what is accomplished by canonical correlation, or canonical analysis. Essentially, the idea is to study the relationship between groups of metric variables by relating low-dimensional projections. When Y reduces to a scalar random variable Y, canonical correlation boils down to multiple linear regression. To illustrate one potential application of canonical correlation, consider a set of marketing activities (advertising effort, packaging quality and appeal, pricing, bundling of products and services, etc.) and a set of corresponding customer behaviors (willingness to purchase, brand loyalty, willingness to pay, etc.); clearly, to apply the approach, we must introduce a metric scale to measure each variable. It is natural to consider the variables in the second set as dependent variables; therefore, this is a case in which we wish to explore dependence, but canonical correlation can also be used to investigate interdependence. In fact, canonical correlation forms the basis for other multivariate analysis techniques.
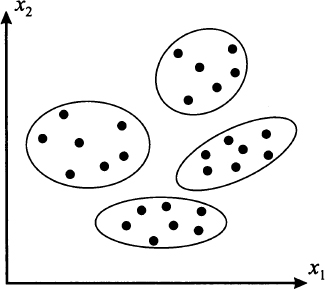
Fig. 15.3 Four bidimensional clusters.
Leave a Reply